

For a small tomographic window, it is difficult for satellite signals to cover all voxels divided in the study area. Therefore, it is necessary to set the time coverage of a water vapor tomographic solution, namely the tomographic window. However, the water vapor content in space changes dynamically, and it is unreasonable to last for an excessively long time for a tomographic solution which can introduce more errors. To realize the water vapor tomographic solution, more satellite signals need to be added to the tomographic model, that is, each water vapor tomographic solution covers a period of time. In these three types of water vapor tomographic methods, the number of voxels which signal rays pass through are always limited in the research region at a certain moment due to the unfavorable geometry of ground GNSS sites and fixed structure of satellites (Guo et al., 2016 Zhao et al., 2018). The Multiplicative Algebraic Reconstruction Techniques (MART) and the Simultaneous Iteration Reconstruction Techniques (SIRT) that belongs to the ART family were also adopted. ( 2011) utilized the ART that processes observation by observation to inverse the tomographic model. Then the adaptive Kalman filtering and the robust Kalman filtering were proposed successively to reconstruct the water vapor information (Jiang et al., 2013 Rohm et al., 2014). Assuming that the Water Vapor Density (WVD) in each voxel follows the Gauss-Markov random walk pattern for a certain period of time, Gradinarsky and Jarlemark ( 2004) constructed the corresponding state equation and established the method of water vapor tomographic solution based on the Kalman filtering. ( 2000) used the Least squares approach to solve the tomographic equations by adding a set of constraints, and the method and its variates are discussed in a lot of literature (Adavi & Mashhadi-Hossainali, 2014 Shafei & Hossainali, 2020). the Least squares, the Kalman filtering, and the Algebraic Reconstruction Technique (ART). In GNSS water vapor tomography, scholars have developed different methods to solve the tomographic model, which can be mainly grouped into three types of methods, i.e. In tomographic approach for water vapor, a study region covered by GNSS sites is divided into a number of voxels, and the Slant Water Vapor (SWV) derived from GNSS observations passing though these voxels are used for estimating the water vapor information. At present, it is one of the most powerful and accurate methods for the reconstruction of three-dimensional water vapor distribution (Sa et al., 2021 Yang et al., 2020a Yao et al., 2017). ( 1999) and then realized by Flores et al. The concept of GNSS water vapor tomography was first proposed by Braun et al. In Global Navigation Satellite System (GNSS) meteorology, water vapor tomography has attracted more and more attention and played increasingly a crucial role since it can accurately obtain the three-dimensional water vapor information (Yang et al., 2022a Zhang et al., 2020 ). It is recommended to set a tomographic window width of 10 min and a sampling rate of 300 s in a GNSS water vapor tomographic experiment with dense GNSS site like Hong Kong. Numerical results show that the tomographic results with the three methods get better as the width of the tomographic window decreases and the sampling rate increases in these 12 schemes, and it is found that the Least squares method is most affected by the two factors, followed by Kalman filtering and MART methods. This study uses Hong Kong as the research area to explore the impact of 12 schemes with different tomographic window and sampling rate on the three water vapor tomography methods, including Least squares, Kalman filtering, and Multiplicative Algebraic Reconstruction Technique (MART). These two factors affect not only the number of available signal rays from the satellites, but also the number of tomographic voxels crossed by the signal rays. As the multi-GNSS and more ground-based GNSS sites can be incorporated into the regional water vapor tomographic model, determining the tomographic window and sampling rate is crucial for the modelling of the water vapor tomography.
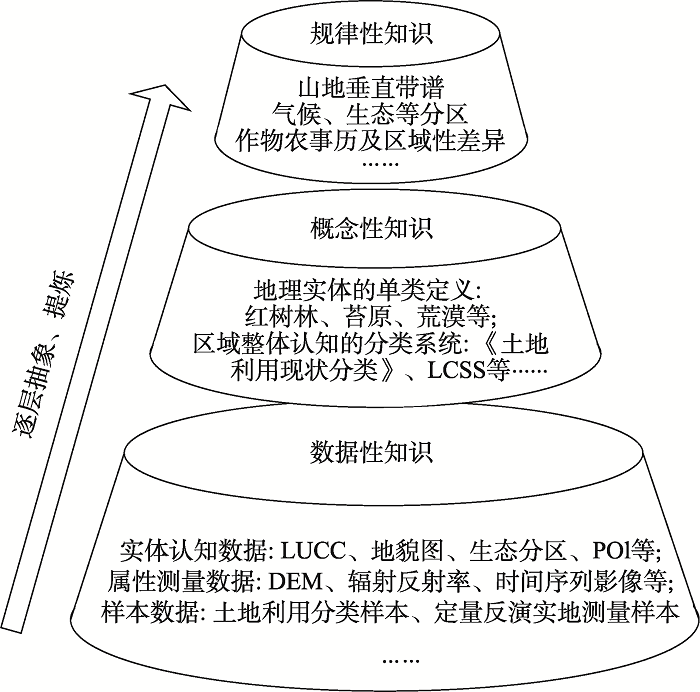
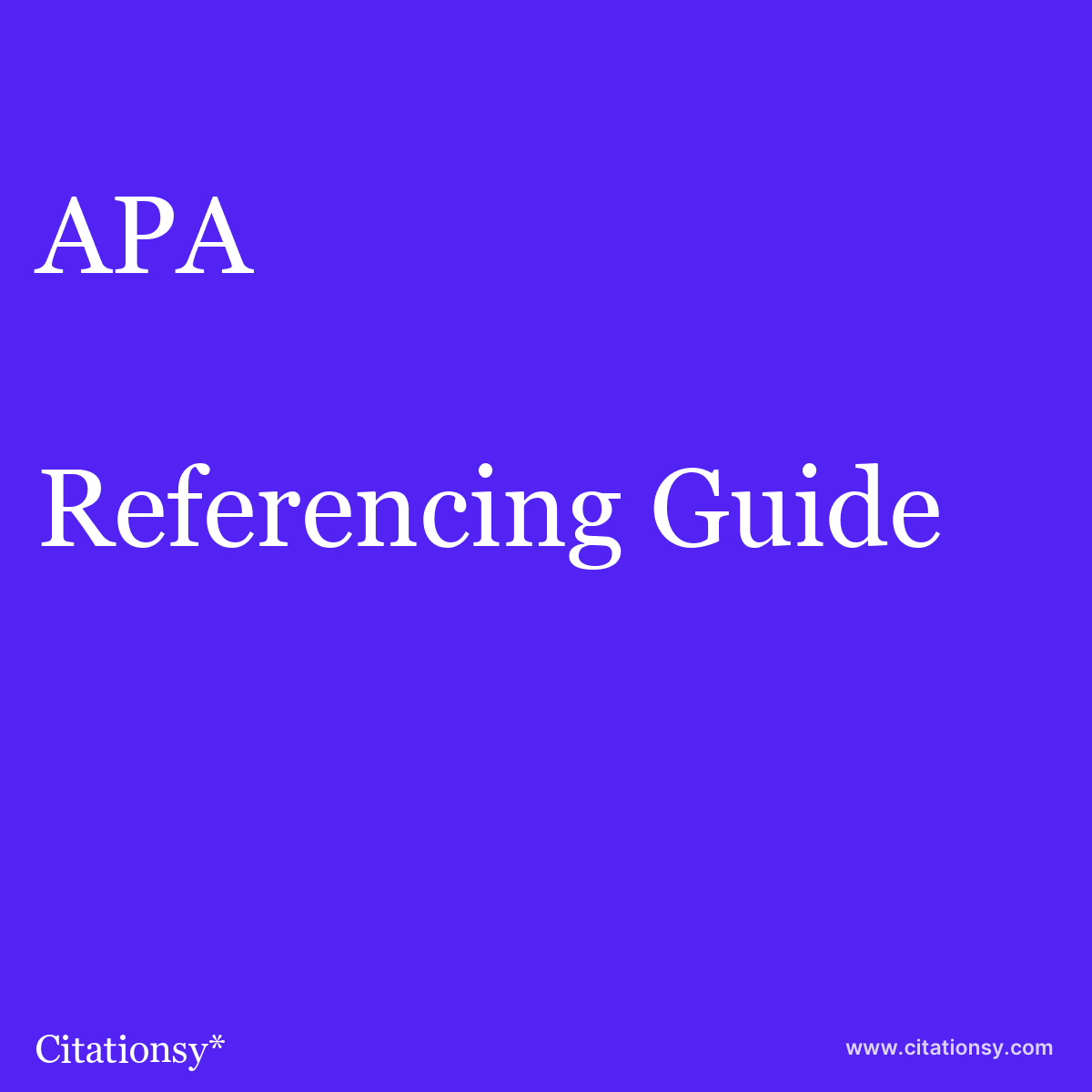

The ground-based Global Navigation Satellite System (GNSS) water vapor tomography is increasingly important in GNSS meteorology.
